
MitoNet and empanada
Empanada (EM Panoptic Any Dimension Annotation) is a tool for panoptic segmentation of organelles in electron microscopy (EM) images in 2D and 3D. Panoptic segmentation combines both instance and semantic segmentation enabling a holistic approach to image annotation with a single deep-learning model. To make model training and inference lightweight and broadly applicable to many EM imaging modalities, Empanada runs all expensive operations on 2D images or run-length encoded versions of 3D volumes.
Direct LinkManuscript on BioRxiv:
BioRxiv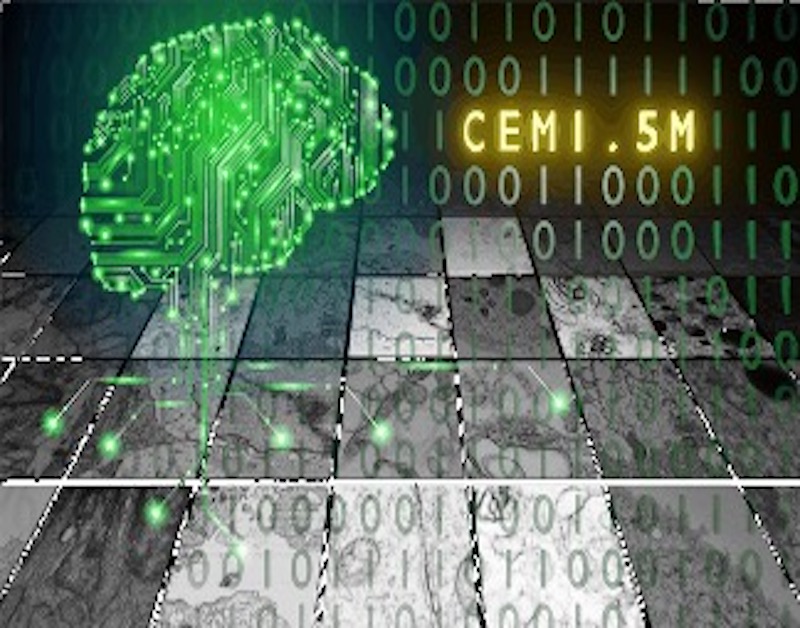
CEM1.5M
A heterogeneous, non-redundant, information-rich, and relevant unlabeled EM image dataset (at ∼1.5 x 106 images, the largest of its kind to our knowledge) for use as a database to pre-train and sample images for mitochondria, or other organelles, segmentation. We used CEM1.5M to pre-train MitoNet
Dataset uploaded at:
Dataset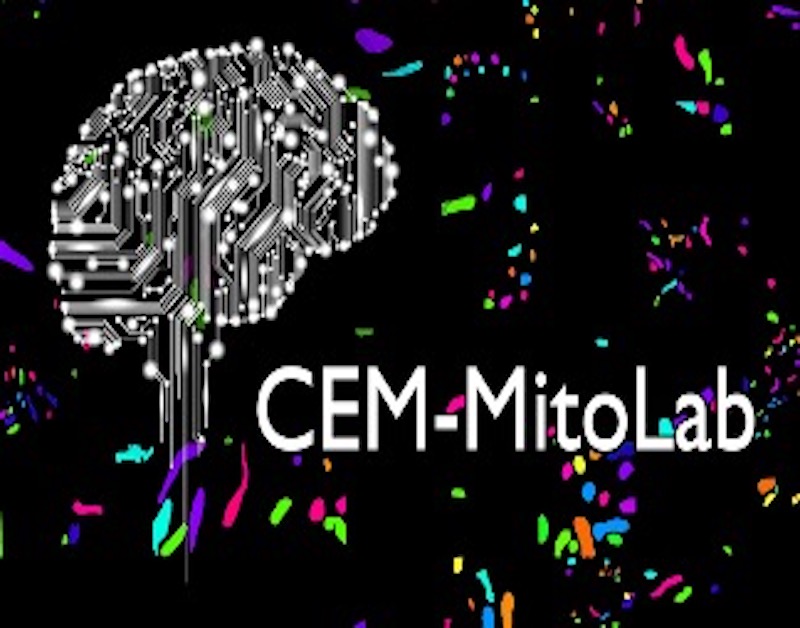
CEM-MitoLab
A dataset of ~22K cellular EM 2D images with label maps of ~135K mitochondrial instances, for deep learning. We used this labeled dataset to train MitoNet.
Dataset uploaded at:
Dataset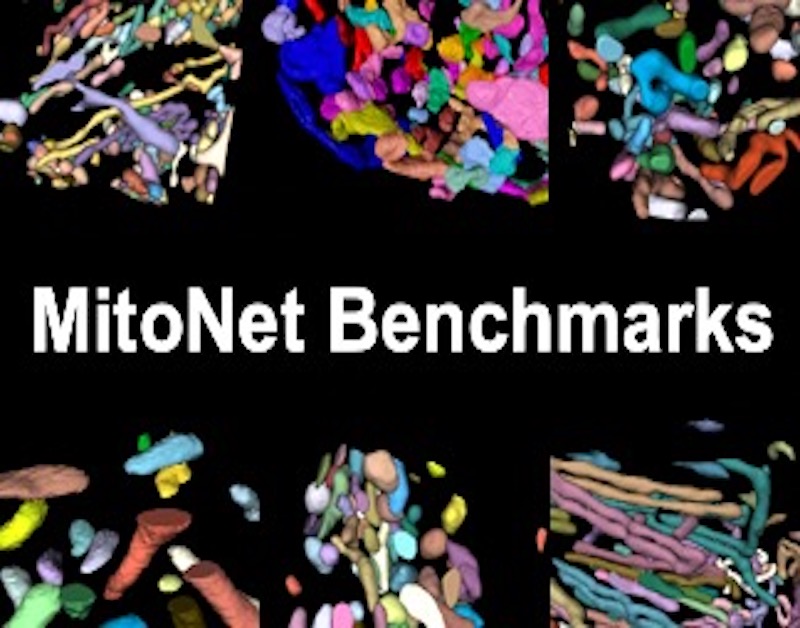
MitoNet Benchmark
7 benchmark datasets with instance labeled mitochondria, used to test MitoNet. 1 dataset is a series or random TEM images; the others are isotropic volume EM (FIB-SEM) data from various biosamples and sample preparation techniques.
Dataset uploaded at:
DatasetPosters
Scroll down this page to watch acompanying movie
Efficient skeleton editing in a VR environment facilitates accurate modeling of highly branched mitochondria
Ryan W Conrad, Thomas Ruth, Falko Löffler, Steffen Hadlak, Sebastian Konrad, Christian Götze, Christopher Zugates, Molly McQuilken and Kedar Narayan
Download
Towards Artifact-free FIB-SEM datasets: Strategies during image acquisition of resin-embedded biological samples
Kedar Narayan, Alexandre Laquerre and Michael Phaneuf
Download
Super-resolution cryo-fluorescence microscopy of high-pressure-frozen thick samples: a screening study of C. elegans
Irene Y Chang, Mohammad M Rahman, Adam Harned, Orna Cohen-Fix and Kedar Narayan
Download Watch Video
Videos
Cryo LM of high-pressure-frozen thick samples (C. elegans)
This video describes a workflow to execute cryo fluorescence microscopy on high-pressure-frozen C. elegans worms. This collaboration with Carl Zeiss GmbH was presented (Poster #1085) at Microscopy & Microanalysis 2020.
“2.5D” Neural Network based segmentation of mitochondria
This video describes a method to enforce prediction consistency across orthogonal planes (hence 2.5D) to improve neural network segmentation of mitochondria in a FIB-SEM image volume. This work was presented (poster #831) at Microscopy & MIcroanalysis 2020.
Towards artifact-free FIB-SEM acquisition
This video describes artifact-free FIB-SEM data acquisition strategies, and accompanied a poster (#535) presented at Microscopy & Microanalysis 2020
Editing mitochondrial skeletons in a virtual reality (VR) environment
In this movie, we describe a module in ArivisVR to import, edit and export corrected mitochondrial skeletons. This work (in collaboration with arivis Inc) was presented (Poster #448) at Microscopy & Microanalysis 2020.