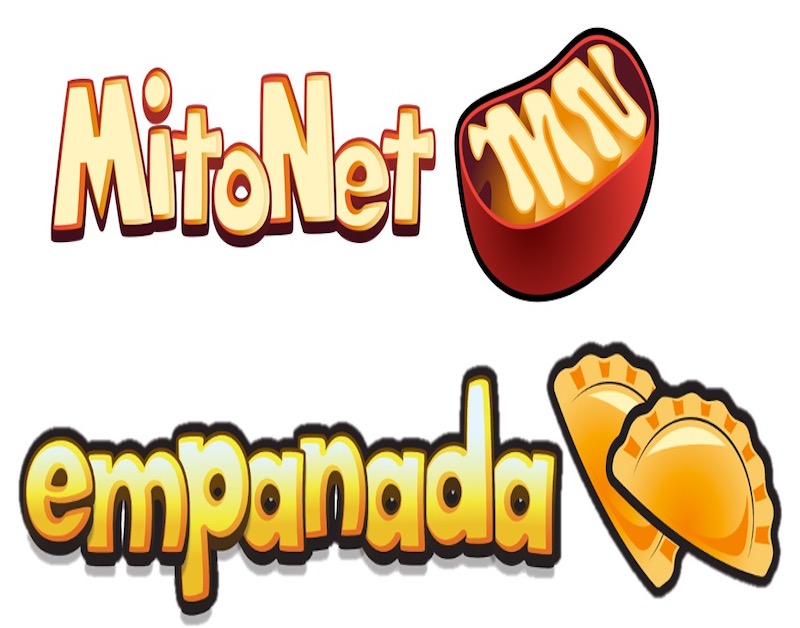
empanada v1.1 now released
empanada and MitoNet
Empanada (EM Panoptic Any Dimension Annotation) is a tool for panoptic segmentation of organelles in electron microscopy (EM) images in 2D and 3D. Panoptic segmentation combines both instance and semantic segmentation enabling a holistic approach to image annotation with a single deep-learning model. To make model training and inference lightweight and broadly applicable to many EM imaging modalities, Empanada runs all expensive operations on 2D images or run-length encoded versions of 3D volumes.
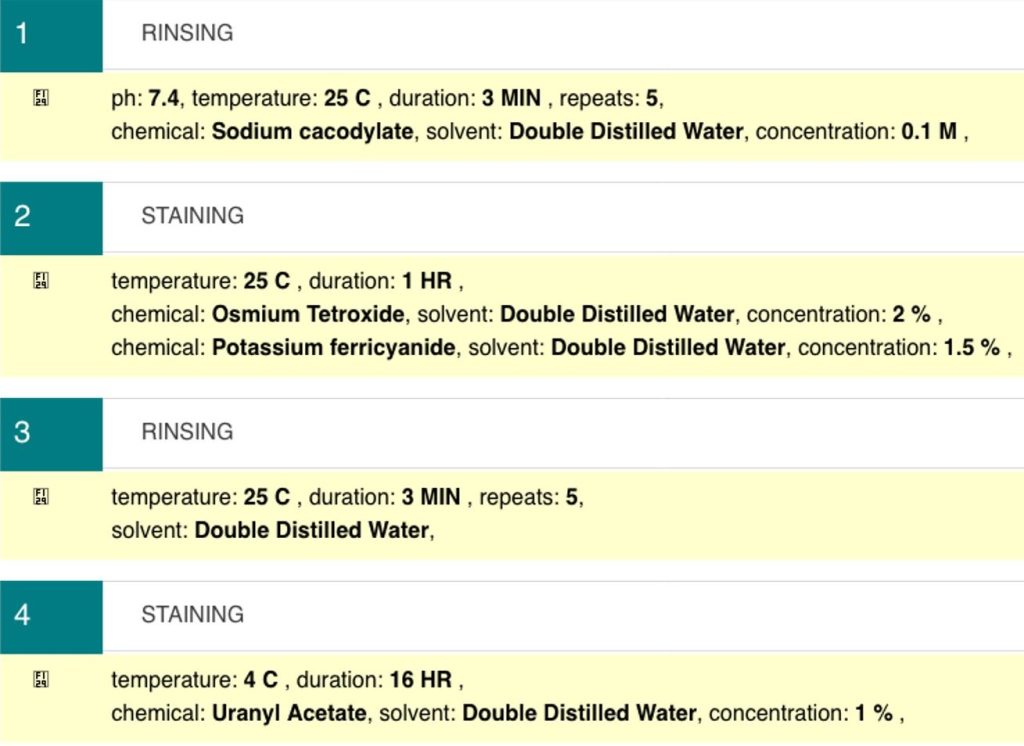
Sample preparation widget
The Sample Preparation Widget (SPW) is an online tool available through the EMPIAR deposition system that is designed to allow easy confirmation of protocols with point-and-click functionality. It uses controlled vocabularies where possible and allows free-text information if required. This tool will be used into the future to show what sample preparation protocols were used to create a vEM dataset that has been deposited to EMPIAR.
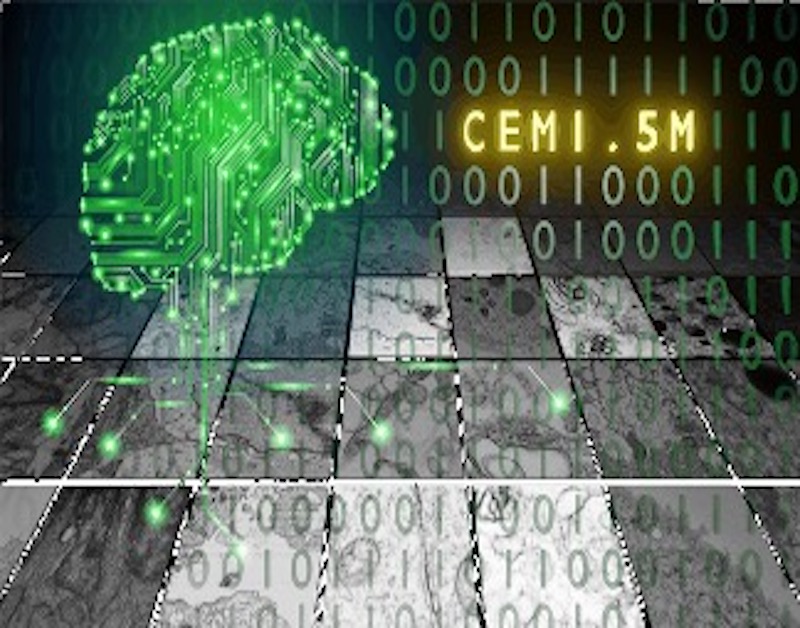
CEM1.5M
A heterogeneous, non-redundant, information-rich, and relevant unlabeled EM image dataset (at ∼1.5 x 106 images, the largest of its kind to our knowledge) for use as a database to pre-train and sample images for mitochondria, or other organelles, segmentation. We used CEM1.5M to pre-train MitoNet.
Dataset uploaded at:
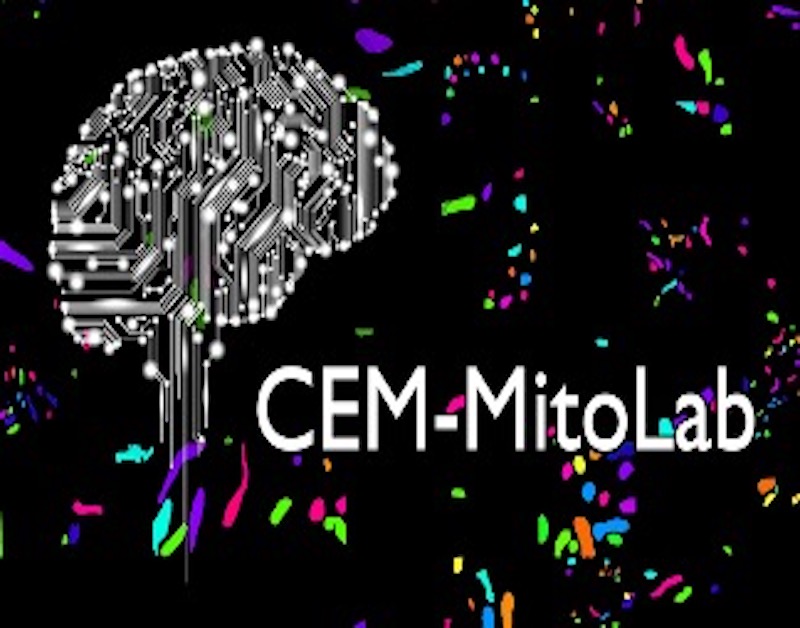
CEM-MitoLab
A dataset of ~22K cellular EM 2D images with label maps of ~135K mitochondrial instances, for deep learning. We used this labeled dataset to train MitoNet.
Dataset uploaded at:
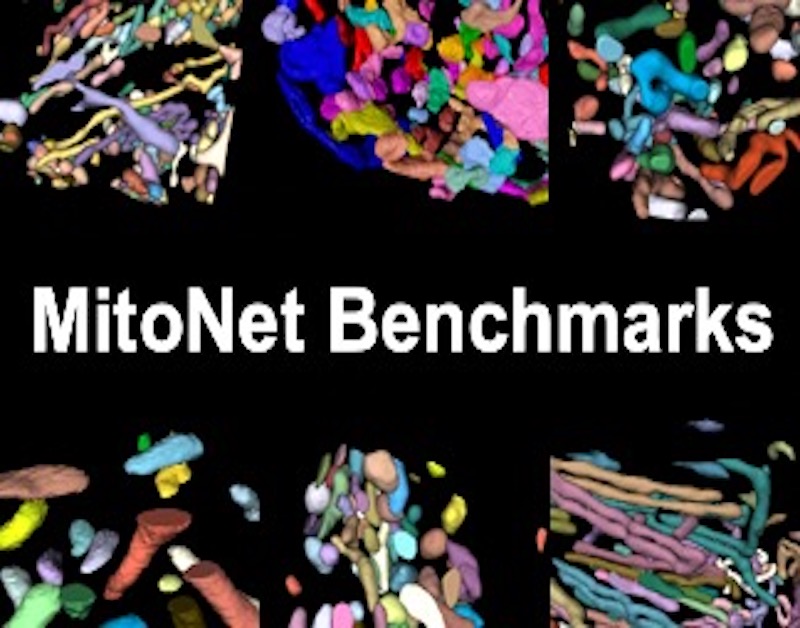
MitoNet Benchmark
7 benchmark datasets with instance labeled mitochondria, used to test MitoNet. 1 dataset is a series or random TEM images; the others are isotropic CvEM (FIB-SEM) data from various biosamples and sample preparation techniques.
Dataset uploaded at:
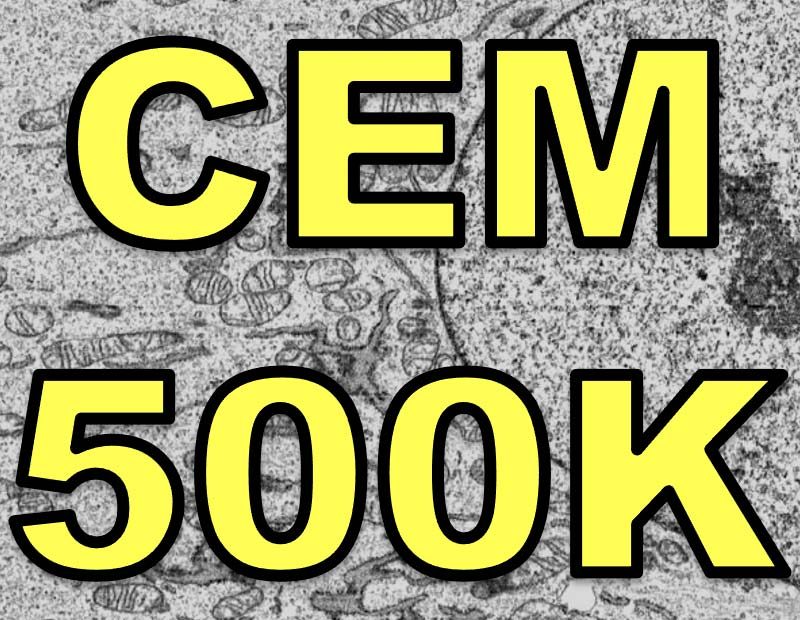
CEM500K
A large-scale heterogeneous unlabeled cellular electron microscopy image dataset for deep learning methods.
Dataset uploaded at:
Full Code Uploaded:
Manuscript on BioRxiv: